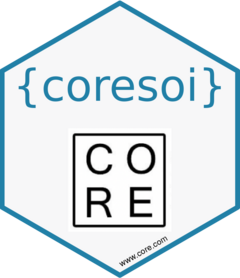
Choice of elementary indicators
Source:vignettes/articles/ChoiceOfElementaryIndicators.Rmd
ChoiceOfElementaryIndicators.Rmd
The strengths and weaknesses of composite indicators (CIs) largely derive from the quality of the underlying elementary indicators, which in our context are the red flags of corruption risk in public procurement. Red flag indicators are at the core of corruption risk assessment systems developed to identify corruption risks and settle effective strategies for mitigating them in a preventative logic. They are proxy measures for corruption, signalling risks of corruption, rather than actual corruption. Red flags are then expected to be correlated with corrupt practices, rather than perfectly matching them (OECD 2019; Mihály Fazekas and Kocsis (2017a) ).
Typically, corruption in public procurement aims at steering the contract to the favoured bidder without detection, in an institutionalised and recurrent fashion. Indeed, institutionalised grand corruption aims primarily at extracting rents. In public procurement, rents can be gained by pre-selecting companies, which then receive extra profit by charging higher than average market prices for the contract object delivery (Abdou et al. 2020). To assess corruption risks by quantifying extra-profit, researchers and policy makers would need data on price and quantity of procured deliveries, which are usually available in most public procurement administrative datasets, but incomparable across time and space. Thus, complementary proxies of corruption risk are proposed, such as the circumstance that a procurement procedure receives a single offer by a single bidder. Indeed, the number of bids submitted, especially when there is only one submitted bid, has been shown to be associated with corruption risks (Klasnja et al. 2015) and extensively adopted in the literature as a proxy of corruption. Other recurrent risk indicators are (Mihály Fazekas and Kocsis 2017b): absence of publication of the call for tenders (when the publication is voluntary); non-open or exceptional procedure types (Auriol, Straub, and Flochel 2016), such as direct awards, which allow to stifle competition and steer contracts to the selected bidders; too short advertisement time-periods, which can inhibit non-connected bidders to develop adequate bids in time; subjective and hard-to-quantify evaluation criteria (in place of price-related criteria), which imply certain discretionary margins and may limit accountability control mechanisms; too short time intervals to award contracts, as snap decisions may imply premediated appraisal.
Such proxy indicators are typically obtained by accounting for the degree of unjustified restriction of competition in the public procurement process (Mihály Fazekas, Tóth, and King (2016); Mihaly Fazekas, Cingolani, and TTth (2016) ). They operationalise the corruption definition according to which corruption works when the principles of open and fair competition are circumvented by public officials, in order to recurrently award public contracts to connected companies (Mihály Fazekas and Kocsis 2017c). The above corruption red flags signal corruption only if competition is expected in the absence of corruption (Mihály Fazekas and Kocsis 2017d). Hence, they cannot be effectively employed when markets are under non-competitive circumstances. Further, and most importantly, under the relaxed public procurement control systems introduced by crises such as the Covid-19 emergency, many of the bids receive a single offer and are adopted through exceptional and very rapid procedures, in order to supply shortages of essential goods. Relying on the above-mentioned red flags under crises would then likely lead us to overestimate corruption risks and to give rise to false positives, as high values are likely to mirror legitimate procedural choices allowed by a relaxed regulatory framework rather (or other) than an actual high level of corruption. Indeed, red flag indicators may suffer from overestimating corruption risks, as there can be non-corrupt instances where red flag indicators signal risk, giving rise to false positives (OECD 2019). In both normal and exceptional circumstances, the distorting effect of false positives can be attenuated by carefully selecting the elementary risk indicators that are most closely associated with other corruption risk signals.
Given the premises above, in the CO.R.E. project we propose to identify companies and contracting authorities which may be at risk of being corrupt across crises, through the selection of a set of red flags, only in part derived by the current literature, but computed through a novel procedure which exploits the time discontinuity introduced by crisis outbreak, that is, the possibility to distinguish two time-spans, a pre-crisis period and a post-crisis period. Specifically, as it will be further clarified in the next sections, the approach compares, whenever possible, company and/or contracting authority behaviours after the emergency outbreak with respect to their historical behaviour and assesses the associated risk through statistical testing. Given the focus on crisis scenarios, each red flag will be calculated regarding contracts awarded in the relevant market for the crisis at issue (i.e., the health market classified with the Common Procurement Vocabulary (CPV) 33 for the Covid-19 crisis).
The proposed procedure is:
- extensible to other crisis contexts to assess the risk of corruption in public procurement by i. setting the two time-spams in accordance with the entry date of the legislative act acknowledging the beginning of the emergency period; ii. selecting the relevant contracts objects (i.e., CPV), depending on the pertinent markets most concerned with the specific crisis at hand;
- replicable to other national contexts where the data needed for the red flag calculation are available;
- adjustable on account of market trends across the crisis, by setting the statistical test hypotheses accordingly.